Artificial Intelligence, or AI, has become a buzzword in recent years, captivating the imagination of individuals, businesses, and industries worldwide. To delve deeper into the concept of AI and comprehend its functioning, it is important to understand what AI is and how it operates.
In this comprehensive guide, we will delve into the intricate world of artificial intelligence, exploring its fundamental concepts, underlying technologies, and real-world applications. By the end of this article, you’ll have a clear understanding of how AI works and its transformative potential.
Understanding Artificial Intelligence
Artificial Intelligence (AI), denoting the replication of human intelligence in machines, is often abbreviated as AI. It encompasses the creation of algorithms and models that empower computers and machines to replicate human cognitive processes, including learning, reasoning, problem-solving, and decision-making. AI systems can analyze extensive datasets, identify patterns, and then provide predictions or recommendations accordingly.
Types of Artificial Intelligence
AI is commonly classified into two primary types:
Narrow or Weak AI:
It is designed for a specific task or domain, and it performs within that limited scope. Examples include virtual personal assistants like Siri, image recognition software, and recommendation systems like Netflix’s algorithm.
General or Strong AI:
It possesses human-like intelligence and the ability to carry out multiple tasks with adaptability and creativity. Achieving true General AI remains a long-term goal of AI research and development.
See Also: How Generative AI will Transform your Business
Core Components of AI
To comprehend how AI works, it’s essential to understand its core components:
- Data: Data is the lifeblood of AI. Machines learn from data, and the quality and quantity of data significantly impact AI performance. Large datasets are fed into AI models to train them, allowing the AI to recognize patterns and make predictions.
- Algorithms: Algorithms are mathematical instructions that tell the AI to process data and make decisions. Machine learning, deep learning, and natural language processing, among others, are all performed using different algorithms.
- Computational power: AI tasks, especially deep learning, require substantial computational power. Graphics Processing Units (GPUs) and specialized hardware like Tensor Processing Units (TPUs) are commonly used to accelerate AI computations.
Machine Learning: The Heart of AI
As a component of AI, machine learning focuses on constructing algorithms that allow machines to acquire knowledge from data and improve their performance. Here’s an explanation of how machine learning operates:
- Data collection: Data can possess structure, such as being organized within databases, or it can remain unstructured, for instance in the form of text or images.
- Data preprocessing: Raw data is often noisy and unorganized. Data preprocessing involves cleaning, transforming, and normalizing the data to make it suitable for training an AI model.
- Model training: During training, the AI model is exposed to the preprocessed data. The algorithm adjusts its internal parameters to identify patterns and relationships within the data.
- Evaluation: Models are tested on new, unseen data after training. To determine if they can make accurate predictions, a separate dataset is used (testing data).
- Deployment: Once the model performs well on the testing data, it can be deployed in real-world applications, making predictions or decisions based on incoming data.
Deep Learning: Subfield Revolutionizing AI
Deep learning has a huge capacity to handle intricate data types like images, audio, and text. It is defined by the utilization of neural networks, which draw inspiration from the structure and functions of the human brain.
- Neural networks: Neural networks consist of interconnected layers of artificial neurons, each with weights and biases. These networks can have multiple hidden layers, allowing them to capture intricate patterns in data.
- Training deep learning models: Training deep learning models involves forward and backward propagation, where the network adjusts its parameters iteratively to minimize the difference between its predictions and the actual target values.
- Convolutional Neural Networks (CNNs): CNNs, also known as Convolutional Neural Networks, are specifically crafted neural networks intended to excel in the domain of image recognition tasks. They use convolutional layers to extract features from images automatically.
- Recurrent Neural Networks (RNNs): RNNs are suitable for sequential data, such as text and speech. They have memory cells that can capture dependencies in sequential data.
Natural Language Processing (NLP)
NLP is a branch of AI dedicated to empowering machines to comprehend, interpret, and produce human language. It is crucial in chatbots, language translation, and sentiment analysis applications.
Tokenization
Tokenization refers to the process of dividing text into smaller entities, such as individual words or phrases, for the purpose of enhancing analysis. This practice is utilized to streamline the evaluation process efficiently.
Word embeddings
Word embeddings represent words as numerical vectors, allowing AI models to understand the semantic relationships between words.
Sentiment analysis
NLP models can determine the sentiment or emotional tone of the text, making them valuable for understanding customer feedback and social media sentiment.
Also Read: How AI Is Revolutionizing the Way We Find Jobs
Reinforcement Learning
Reinforcement learning is a subset of machine learning where agents learn to make a sequence of decisions to maximize a reward. It is commonly used in applications like autonomous robotics and game-playing AI.
Agent and Environment
In the domain of reinforcement learning, an agent engages in dynamic interactions with an environment. The agent takes actions, receives feedback (rewards or penalties), and learns to optimize its actions over time.
Exploration vs. Exploitation
Reinforcement learning involves a trade-off between exploration (trying new actions) and exploitation (choosing actions with known rewards). Agents must strike a balance to maximize long-term rewards.
Real-world Applications of AI
AI has found applications in various industries, transforming the way businesses operate and improving our daily lives. Some notable applications include:
- Healthcare: AI is harnessed to facilitate disease diagnosis, expedite drug discovery, and devise tailored treatment plans.
- Finance: AI is utilized within the financial industry to enhance fraud detection capabilities, facilitate algorithmic trading strategies, and bolster risk assessment processes.
- Transportation: AI is capable of powering self-driving vehicles, managing traffic effectively, and enabling predictive maintenance for transportation infrastructure.
- Retail: Retailers use AI for inventory management, demand forecasting, and personalized recommendations.
See Also: Google Bard Alternatives
Ethical Implications of AI
While AI holds great promise, it also raises ethical concerns. It is essential to consider issues like bias in AI algorithms, job displacement, and data privacy as AI advances.
Bias and fairness
AI algorithms have the potential to acquire biases from the training data they are exposed to, resulting in outcomes that may be unfair or discriminatory. Intensive endeavors are underway to create AI systems that are more equitable and free from bias.
Job displacement
As AI continues to automate specific tasks, the issue of job displacement becomes a significant concern. New job opportunities can also be created by AI, however.
Data privacy
There are concerns about privacy and security because this technology relies on huge amounts of data. Regulations such as GDPR have the main objective of safeguarding the personal data of individuals.
The Future of AI
The field of AI is constantly evolving, with ongoing research and advancements. The future of AI holds exciting possibilities, including:
AGI – The Pursuit of General AI
Researchers continue to work towards achieving General AI, which would have the intelligence and adaptability of human intelligence. Achieving AGI would mark a profound leap in AI capabilities and could revolutionize various industries.
Explainable AI (XAI)
As AI systems become more complex, transparency and interpretability become more important. Explainable AI (XAI) seeks to make AI decisions understandable to humans, enhancing trust and accountability.
AI in Education
AI-powered educational tools can provide personalized learning experiences, helping students of all ages acquire knowledge more efficiently.
AI in Climate Change Mitigation
AI analyzes climate data, optimizes energy consumption, and develops sustainable solutions to combat climate change.
AI in Healthcare Innovation
AI is poised to continue significantly contributing to healthcare, from early disease detection to drug discovery and telemedicine.
Also Read: 5 ChatGPT Alternatives for Writing
Conclusion
Artificial Intelligence is a groundbreaking field that has the potential to reshape the way we live and work. AI’s real-world applications span numerous sectors, from healthcare and finance to transportation and retail. However, the ethical implications of AI, such as bias and job displacement, must be carefully addressed.
Looking ahead, the future of AI holds the promise of achieving greatness, making AI systems more explainable and transparent. However, as AI advances, society must navigate these developments with a commitment to ethical and responsible deployment, ensuring that the benefits are accessible and equitable for all.
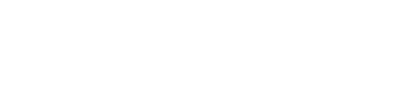