In today’s rapidly evolving landscape, companies are looking at new ways to gain a competitive edge in the market. As marketers, the onus is on us to understand our audience better and craft messages that resonate with them. One of the tools that has significantly revolutionized this process is Artificial Intelligence (AI). Notably, AI plays a vital role in predictive analysis, empowering businesses to deliver top-notch targeted marketing campaigns that yield impressive returns.
But which are these roles played by AI in predictive analysis? This article sheds light on the pivotal role AI plays in refining marketing strategies through predictive analysis.
Understanding Predictive Analysis
Before we delve into the role of AI in predictive analysis, it’s essential to understand what predictive analysis entails. Predictive analysis involves using historical data to predict future outcomes. While it has been around for years, the availability of data (thanks to the internet) and advancements in computing power have accelerated its application in marketing.
For instance, consider how the intricacies of AI-driven tools in finance, such as MTD VAT software, invoicing software, and more, have streamlined accounting processes for businesses. Similarly, in predictive analysis, AI helps break down the complexities of consumer behavior to deliver optimal results. From forecasting marketing trends to predicting consumer buying habits, predictive analytics offers a tantalizing glimpse into the future.
The Role of AI in Predictive Analysis for Targeted Marketing
1. Unraveling the Layers of Consumer Data
Every interaction a consumer has online, from clicking any webpage to making a purchase, data is generated. The sheer volume of this data makes it hard for any human to analyze and derive insights without assistance. This is where AI comes in. Through sophisticated algorithms, AI can go through massive datasets, identifying trends and patterns that can be invaluable for marketers. This saves marketers the hassle of looking into large volumes of data, creating more time for them to focus on other tasks.
2. Enhancing Personalization
Predictive analysis driven by AI allows for a deeper understanding of individual consumer preferences. By anticipating what a consumer might want or need next, businesses can tailor their messaging accordingly. This personalization can manifest in various ways, from product recommendations on an e-commerce site to curated content on a streaming platform.
Related: How AI Will Make the Marketing Mix More Personalized?
3. Optimizing Marketing Budgets
Through predictive analysis, AI can also forecast the potential success of different marketing strategies. Marketers can determine which campaigns are likely to yield the highest ROI, allowing for more strategic allocation of budgets. By pinpointing the most effective channels and tactics, businesses can maximize their marketing spend.
4. Real-time Decision Making
In a fast-paced digital environment, being able to make swift decisions can be a game-changer. AI enables real-time analysis, allowing businesses to adjust their marketing strategies on the fly based on immediate feedback. This adaptability can be especially crucial during time-sensitive campaigns or in response to unforeseen events.
5. Future Projections and Long-term Strategies
Beyond immediate campaigns, AI’s predictive capabilities can also shed light on longer-term trends. Businesses can get a glimpse into future market conditions, helping them align their long-term strategies with projected consumer needs and preferences.
See Also: Calendly Alternatives
Predictive Analytics in Marketing Examples
Predictive analytics in marketing involves using historical and real-time data to forecast future trends and behaviors, helping marketers make informed decisions. Here are some examples of how predictive analytics can be applied in marketing:
- Customer Lifetime Value (CLV) Prediction: By analyzing past purchasing behaviors, engagement patterns, and demographics, predictive analytics can estimate the potential value a customer may bring to a business over their lifetime. This information helps marketers allocate resources more effectively, focusing on high-value customers.
- Churn Prediction: Predictive models can analyze customer behaviors to identify those at risk of churning (discontinuing the use of a product or service). For instance, a subscription-based streaming service can predict users’ likely to cancel their subscriptions based on reduced engagement and offer targeted retention campaigns to keep them engaged.
- Lead Scoring and Conversion Prediction: By assessing historical data on leads and conversion patterns, predictive analytics can assign scores to leads based on their likelihood to convert into customers. This enables sales teams to prioritize leads and customize their outreach strategies accordingly.
- Product Recommendation Engines: E-commerce platforms utilize predictive algorithms to suggest products to customers based on their browsing and purchasing history. For example, Amazon’s “Customers who bought this also bought…” feature uses predictive analytics to recommend complementary products.
- Inventory Management: Retailers can predict future demand for products by analyzing historical sales data, seasonal trends, and external factors. This helps optimize inventory levels and prevent stockouts or overstock situations.
- Dynamic Pricing: Airlines and ride-sharing services often adjust prices based on demand and other factors. Predictive models analyze historical data to predict when demand will be high and adjust prices accordingly.
- Email Marketing Optimization: Predictive analytics can determine the best time to send emails to maximize open and click-through rates. By analyzing past recipient behaviors, marketers can tailor their email campaigns for optimal engagement.
- Content Personalization: Media and content platforms can use predictive analytics to recommend articles, videos, or other content to users based on their past preferences, increasing user engagement and time spent on the platform.
- Social Media Engagement Prediction: Marketers can use historical data on social media engagement to predict which types of content are likely to perform well. This helps in creating more engaging posts and optimizing content strategies.
- Event Attendance Prediction: Organizations hosting events can use predictive analytics to estimate attendance based on historical event data, demographics, and other relevant factors. This helps in planning logistics and marketing efforts.
Predictive analytics empowers marketers to make data-driven decisions, optimize resource allocation, and create more personalized and effective campaigns, ultimately leading to better customer experiences and improved business outcomes.
See Also: Google Bard Alternatives
Conclusion
The fusion of AI and predictive analysis is reshaping the marketing landscape. As AI continues to advance, its integration into marketing strategies will become more seamless, offering businesses unprecedented insights into consumer behavior.
Companies that embrace this synergy will not only create more effective marketing campaigns but will also foster stronger connections with their customers, ensuring sustained growth and success in the competitive market. If you have not yet incorporated this promising synergy into your business, don’t wait any longer!
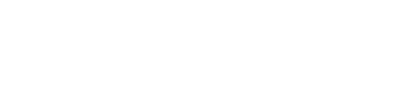