In the dynamic world of finance, where the pace of change is as rapid as the data it generates, financial institutions and enterprises face a constant challenge: making accurate and timely business loan decisions. For credit card companies, lenders, advisory firms, and other large entities focused on small business lending in the United States, this challenge is compounded by the need for precision and risk mitigation.
Enter the era of data science and machine learning — revolutionary tools that are reshaping the landscape of business loan decisions. This article delves into how these technologies are not just technological enhancements but strategic imperatives, driving smarter, faster, and more reliable lending processes.
The Business Edge of Data Science in Risk Assessment
Risk assessment is a critical part of financial decision-making. Traditional methods, while effective, are limited in handling the complexity and volume of data in the modern financial world. This is where data science steps in, offering a way to sift through massive datasets, identify patterns, and predict outcomes with higher accuracy.
For instance, in underwriting — the process of evaluating a borrower’s risk profile — data science enables firms to analyze vast amounts of data. This includes not just financial records but also external data such as market trends and consumer behavior. The outcome? A more comprehensive risk assessment leads to smarter, safer lending decisions.
Machine Learning: Smartening Loan Decisions
Machine learning, a subset of data science, refines this process further. By employing algorithms that learn from data, financial institutions can predict creditworthiness with greater precision. Incorporating external data sources significantly enhances the precision and effectiveness of risk assessments in the loan decision-making process.
For example, Enigma is a leading provider of business data technology in the United States. Enigma SBA Loans data includes a wealth of information on over 16 million card-accepting U.S. businesses. This data encompasses aspects like revenue growth, financial health, loan history, and credit health, which are crucial in understanding a business’s credit profile.
By integrating such comprehensive data, lenders can gain a more detailed and nuanced understanding of each business. This enhanced understanding enables them to make more informed decisions, tailor their services to meet specific business needs, and navigate the complex landscape of financial lending with greater insight and confidence.
The Ripple Effects Beyond Credit Risk
The impact of data science and machine learning extends beyond assessing credit risk. In areas like anti-money laundering, these tools enhance the detection of suspicious activities, improving security and compliance.
In market risk management, they enable better simulation and scenario analysis, helping financial firms prepare for various market conditions.
The Process: How It Works in Simple Terms
Data science and machine learning tools collect and analyze data from various sources. They then use this data to create models that can predict outcomes — like a borrower’s likelihood of repaying a loan. These predictions help lenders make informed decisions quickly, reducing the time and cost involved in the lending process.
The Benefits for Financial Institutions
The advantages for financial institutions are manifold. Firstly, there’s the aspect of improved decision-making. Accurate predictions mean a lower risk of loan defaults, translating to healthier portfolios and better financial outcomes.
Secondly, the efficiency gained through these technologies enables faster loan processing, enhancing customer satisfaction and competitive advantage.
Conclusion
In conclusion, data science and machine learning are not just technological tools; they are strategic assets for financial institutions. By harnessing these technologies, lenders can not only make smarter loan decisions but also enhance their overall operational efficiency and competitiveness in a rapidly evolving financial landscape. This integration is a testament to how technology and business objectives can align to create a more robust, responsive, and responsible financial sector.
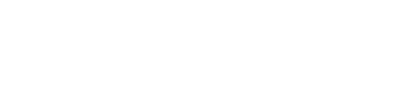